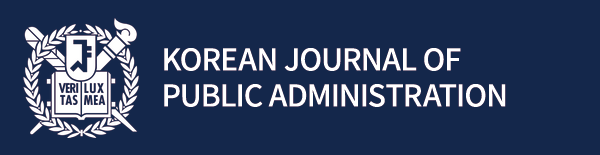
인공지능과 로봇기술의 노동시장 파급효과 분석을 위한 일자리 대체가능성 지수의 개발
초록
인공지능과 4차 산업혁명으로 인해 일자리가 크게 영향을 받을 것으로 전망된다. 특히 인공지능과 4차 산업혁명은 인지적, 비반복적 업무까지 자동화하고 있다는 점에서 구분된다. 그러한 사실에도 불구하고, 기존의 연구들은 대부분 비인지적, 반복적 업무가 루틴화를 통해 대체되었다는 사실을 주목하여 밝히고 있다. 본 연구에서는 이러한 선행연구의 한계를 극복하기 위해, 인공지능과 로봇기술이 함께 작용하는 미래 노동시장의 변화를 보다 종합적으로 분석하고자 하였다. 이를 위해 직무 수행에 필요한 기술과 역량 수준을 제시하는 미국의 ONET 데이터와 인공지능과 로봇기술이 해당 기술과 역량을 얼마나 대체할 수 있을지에 대한 전문가 설문 평가를 바탕으로 직종별 일자리 대체가능성 지수를 도출하였다. 그리고 이를 바탕으로 우리나라 노동시장의 변화와 현황, 그리고 향후 예상되는 파급효과 등을 분석하였다. 분석 결과, 우리나라 노동시장의 절반 이상의 일자리가 심각한 대체 위협에 직면해 있으며, 지난 10년간 대체 수준이 높은 일자리의 비중이 감소하는 추세인 것으로 확인되었다. 반면, 대체가 어려운 일자리의 경우 노동시장의 비중은 가장 적지만, 점차 증가하는 경향을 보였다. 이러한 분석 결과는 직종 간 노동시장의 수급 불균형을 해소하고, 직업 간 전환을 용이하게 하는 제도의 강화와 같은 정책적 노력의 중요성을 시사하였다.
Abstract
The impact of artificial intelligence and the Fourth Industrial Revolution on employment is projected to be profound. These technologies are distinguished by their ability to automate not only routine and non-cognitive tasks but also cognitive and non-routine tasks. However, existing research has predominantly focused on the automation of routine non-cognitive tasks. This study aims to address the limitations of prior research by offering a more comprehensive analysis of the future labor market taking into account the combined effects of artificial intelligence and robotics technologies.
To achieve this, the study utilizes ONET data from the United States. This outlines the abilities and skills required for various job roles and additionally incorporates expert survey evaluations of how robotics and artificial intelligence can replace these skills and abilities, thereby deriving an AI job replacement index for each occupation. The study analyzes the anticipated changes and current status of the labor market in South Korea as well as potential future impacts based on this index.
The analysis reveals that over half of the jobs in the South Korean labor market are at risk of being replaced. Furthermore, there has been a declining trend in the proportion of jobs with high replacement potential over the past decade. Conversely, while jobs that are difficult to replace represent the smallest share of the labor market, their proportion has gradually increased. The findings of this study highlight the importance of policy efforts to mitigate labor market imbalances between occupations and facilitate job transitions, such as strengthening mechanisms that ease occupational shifts.
Keywords:
artificial intelligence, fourth industrial revolution, AI job replacement index키워드:
인공지능, 4차 산업혁명, 일자리 대체가능성 지수Acknowledgments
본 논문은 한국개발연구원의 지원을 받아 수행된 “인공지능과 4차 산업혁명의 노동시장 파급효과와 대안적 복지정책 수단의 효과 분석”의 최종결과물의 일부를 수정 보완하여 작성하였음.
References
- 김민영・조민지・임업. (2017). 자동화 기술의 발전이 지역노동시장 중간일자리 감소에 미치는 영향: 잠재성장모형의 적용. 「국토연구」, 93: 25-41.
- 김세움. (2015). 「기술진보에 따른 노동시장 변화와 대응」. 세종: 한국노동연구원.
- 김세움・고선・조영준. (2014). 「기술진보의 노동시장에 대한 동태적 영향」. 세종: 한국노동연구원.
- 엄상민・정규철. (2021). 「코로나 위기가 초래한 고용구조 변화와 향후 전망」. KDI 경제전망, 2021 하반기. 세종: 한국개발연구원.
- 전병유・정준호・장지연. (2022). 인공지능 (AI) 의 고용과 임금 효과. 「경제연구」, 40(1): 133-156.
- 조지용・박태영. (2013). 잠재 집단분석을 이용한 퇴직자의 사회적 관계유형 변화에 관한 종단적 연구. 「한국가족복지학」, 18(4): 599-623.
- 한지우・오삼일. (2023). 「AI와 노동시장 변화」. BOK 이슈노트 No.2023-30. 서울: 한국은행.
- 홍사흠・유현아・김명한. (2022). 「기술진보가 지역일자리에 미치는 영향: 대체될 것인가? 지속될 것인가?」. 세종: 국토연구원.
-
Acemoglu, D. (1999). Changes in unemployment and wage inequality: An alternative theory and some evidence. American Economic Review, 89(5): 1259-1278.
[https://doi.org/10.1257/aer.89.5.1259]
-
Autor, D. H., & Dorn, D. (2013). The growth of low-skill service jobs and the polairzation of the U.S. labor market. American Economic Review, 103(5): 1553-1597.
[https://doi.org/10.1257/aer.103.5.1553]
-
Autor, D. H., Katz, L. F., & Kearney, M. S. (2006). The polarization of the U.S. labor market. American Economic Review, 96(2): 189-194.
[https://doi.org/10.1257/000282806777212620]
-
Autor, D. H., Levy, F., & Murnane, R. J. (2003). The skill content of recent technological change: An empirical exploration. The Quarterly journal of economics, 118(4): 1279-1333.
[https://doi.org/10.1162/003355303322552801]
-
Damioli, G., Van Roy, V., Vertesy, D., & Vivarelli, M. (2023). AI technologies and employment: Micro evidence from the supply side. Applied Economics Letters, 30(6): 816-821.
[https://doi.org/10.1080/13504851.2021.2024129]
- Felten, E. W., Raj, M., & Seamans, R. (2019). The occupational impact of artificial intelligence: Labor, skills, and polarization. New York NYU Stern School of Business. Available at SSRN: https://ssrn.com/abstract=3368605
- Frey, C. B., & Osborne, M. A. (2013). The future of employment: How susceptible are jobs to computerisation? Working Paper, Oxford Martin School, Programme on the Impacts of Future Technology, University of Oxford.
-
Frey, C. B., & Osborne, M. A. (2017). The future of employment: How susceptible are jobs to computerisation?. Technological forecasting and social change, 114: 254-280.
[https://doi.org/10.1016/j.techfore.2016.08.019]
- Furman, J., Holdren, J. P., Muñoz, C., Smith, M., & Zients, J. (2016). Artificial Intelligence, Automation, and the Economy. Washington, D.C.: Executive Office of the President, The White House.
-
Goos, M., Manning, A., & Salomons, A. (2009). Job polarization in Europe. American Economic Review: Papers and Proceedings, 99(2): 58-63.
[https://doi.org/10.1257/aer.99.2.58]
-
Hickendorff, M., Edelsbrunner, P. A., McMullen, J., Schneider, M., & Trezise, K. (2018). Informative tools for characterizing individual differences in learning: Latent class, latent profile, and latent transition analysis. Learning and Individual Differences, 66, 4-15.
[https://doi.org/10.1016/j.lindif.2017.11.001]
- IBM. (2022). IBM global AI adoption index 2022. IBM Corporation.
- IFR. (2022). World robotics 2022. IFR International Federation of Robotics.
-
Knoblach, M., & Stockl, F. (2020). What determines the elasticity of substitution between capital and labor? A literature review. Journal of Economic Surveys, 34(4): 847-875.
[https://doi.org/10.1111/joes.12366]
- McKinsey & Company. (2017). A future that works: automation, employment, and productivity. Mckinsey Global Institute.
-
Nylund-Gibson, K., & Choi, A. Y. (2018). Ten frequently asked questions about latent class analysis. Translational Issues in Psychological Science, 4(4): 440.
[https://doi.org/10.1037/tps0000176]
-
Spurk, D., Hirschi, A., Wang, M., Valero, D., & Kauffeld, S. (2020). Latent profile analysis: A review and “how to” guide of its application within vocational behavior research. Journal of vocational behavior, 120: 103445.
[https://doi.org/10.1016/j.jvb.2020.103445]
-
Tein, J. Y., Coxe, S., & Cham, H. (2013). Statistical power to detect the correct number of classes in latent profile analysis. Structural equation modeling: a multidisciplinary journal, 20(4): 640-657.
[https://doi.org/10.1080/10705511.2013.824781]
-
Tschang, F. T., & Almirall, E. (2021). Artificial intelligence as augmenting automation: Implications for employment. Academy of Management Perspectives, 35(4): 642-659.
[https://doi.org/10.5465/amp.2019.0062]
-
Webb, M. (2019). The impact of artificial intelligence on the labor market. Unpublished manuscript. Stanford Univ. CA. Available at SSRN: https://ssrn.com/abstract=3482150
[https://doi.org/10.2139/ssrn.3482150]
-
Wright, E. O., & Dwyer, R. E. (2003). The patterns of job expansions in the United States: A comparison of the 1960s and 1990s. Socio-Economic Review, 1: 289-325.
[https://doi.org/10.1093/soceco/1.3.289]
- 조선비즈. “트레이더 600명에서 2명으로…IT 기업된 골드만삭스”, 2017.2.22.